Accurate gene-regulatory inference from pseudotime-lagged causality
Identifying the causal interactions in gene-regulatory networks requires an accurate understanding of the time-lagged relationships between transcription factors and their target genes. Here we describe a convolutional neural network for the inference of gene-regulatory relationships across pseudotime-ordered single-cell trajectories. We show that combining supervised deep learning with joint-probability matrices of pseudotime-lagged trajectories allows the network to overcome important limitations of ordinary Granger causality-based methods, such as the inability to infer cyclic relationships, for example feedback loops. The network outperforms several common methods for inferring gene regulation and predicts novel regulatory networks from scRNA-seq and scATAC-seq datasets given partial ground-truth labels. To validate this approach, we used the technique to identify important genes and modules in the regulatory network of auditory hair cells, as well as likely DNA-binding partners for two hair cell cofactors, Hist1h1c and Ccnd1, and a novel binding sequence for the hair cell-specific transcription factor Fiz1.
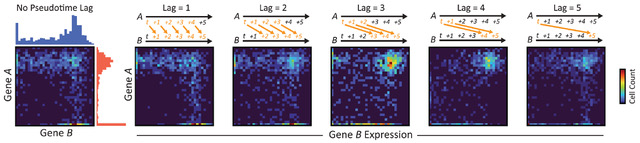